Table of Contents
- Products at a Glance
- How we chose the best GPU for Deep Learning
- Deep Learning Overview
- Things to Consider
- Frequently Asked Questions
- Conclusion
Products at a Glance
1. ASUS ROG Strix RTX 4090 OC
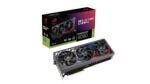
- Pros:
- Incredibly powerful
- Tonnes of VRAM
- Leading CUDA cores
- Cons:
- Power hungry
- Pricey
- Price: Check on Amazon: https://amzn.to/49IN75e
2. ASUS Dual GeForce RTX 4070 Super OC Edition

- Pros:
- Aggressive pricing
- 20% bump in CUDA cores
- Clocked faster than base RTX 4070
- Cons:
- Won’t be able to be pushed too far
- Price: Check on Amazon: https://amzn.to/3TtlDus
3. GIGABYTE AORUS GeForce RTX 4080 Super
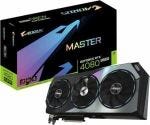
- Pros:
- Sub-$1,000 price tag
- 16GB GDDR6X
- Powerful performance
- Cons:
- The RTX 4090 is faster
- Price: Check on Amazon: https://amzn.to/3V7UrTh
4. ASUS TUF Gaming Nvidia GeForce RTX 3090 OC
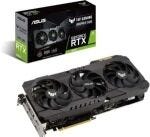
- Pros:
- 24GB GDDR6X memory
- Still powerful in 2024
- Often found discounted from major retailers
- Cons:
- Previous generation
- Somewhat limited availability
- Price: Check on Amazon: https://amzn.to/4c845vi
How we chose the best GPU for Deep Learning
Our selections are based on a combination of factors, including general availability, cost-effectiveness, and the capability for Deep Learning tasks. We focused on Ada graphics cards, considering both current-generation options and included an Ampere option for those with budget constraints. Nvidia’s RTX GPUs, known for their prowess in Deep Learning, were prioritized, ensuring options for various budgets.
Deep Learning Overview
Deep Learning encompasses various fields, from large-scale storage to data science and analytics. Whether you are interested in Nvidia or AMD GPUs, our guide covers models suitable for different tasks within the realm of precision computing. From AMD to Nvidia RTX models, we’ve got you covered.
Things to Consider
Deep Learning involves handling large datasets and advanced calculations using GPU memory and CUDA cores. Nvidia cuDNN library, parallel computing, and FLOPs (floating point operations) play pivotal roles in Deep Learning algorithms. Libraries like Pytorch, Tensorflow, Keras, Pandas, and NumPy leverage GPU chips for Deep Learning inference, where training is implemented.
Frequently Asked Questions
1.Is RTX better than GTX for Deep Learning?
Yes, RTX GPUs are superior to GTX predecessors due to Ada architecture’s improvements, higher VRAM, and Tensor cores, leading to increased power efficiency.
2.Is the RTX 4090 good for Deep Learning?
Certainly, the RTX 4090 stands out as an excellent choice for Deep Learning with its 24GB GDDR6X VRAM, numerous CUDA cores, and large memory bus, making it suitable for single-GPU setups.
3.How do GPUs assist in Deep Learning?
GPUs contribute to Deep Learning by handling extensive data, constructing large neural networks, and performing advanced calculations on large datasets. This machine-assisted mathematics involves parallel computing, matrix operations, and FLOPs.
Conclusion
For the best GPU for Deep Learning in 2024, the ASUS ROG Strix RTX 4090 OC takes the top spot. With its immense VRAM, powerful performance, and competitive pricing, it stands as a solid choice for various AI tasks. Explore our recommendations to find the GPU that aligns with your budget and requirements.
Find the best GPU for Deep Learning in 2024 with our top picks. Discover powerful Nvidia models, including the ASUS ROG Strix RTX 4090 OC, tailored for AI workloads.